Explores the key differences between Data Lakes and Data Warehouses, helping businesses decide which data storage solution best fits their needs. By comparing their architectures, use cases, challenges, and growth trends, it provides insights on optimizing data management for analytics, cost-efficiency, and business intelligence.
Table of Contents
- Understanding Data Management in Modern Businesses
- What is a Data Lake?
- What is a Data Warehouse?
- Data Lake vs. Data Warehouse at a Glance
- When to Choose a Data Lake
- When to Choose a Data Warehouse
- Hybrid Approach: Leveraging Both Data Lake and Data Warehouse
- Factors to Consider When Choosing Between Data Lake and Data Warehouse
- Key Characteristic: Data Lake and Data Warehouse
- Key Challenges of Data Storage
- Conclusion: Finding the Right Data Solution for Your Business
- FAQs
In today’s data-driven world, businesses are dealing with an overwhelming amount of data. Efficient data architecture is crucial for storing, retrieving, and managing data to drive superior transactional and analytical outcomes. Without the right infrastructure, it’s impossible to fully harness the value of your data assets. Choosing between a Data Lake and a Data Warehouse, or even combining both under a unified architecture, is a significant decision that depends largely on the nature of your data and its intended use.
As the demand for data management solutions grows, so does the market. According to a report by Research and Markets, the global Data Lake market is projected to grow from USD 7.9 billion in 2019 to USD 20.1 billion by 2024, at a CAGR of 20.6%. Similarly, the Data Warehouse market is set to expand at a rate of 22.3% through 2026. These architectures cater to different business needs and understanding the right solution is critical for efficient operations, cost optimization, and gaining valuable insights.
In this article, we will explore the differences between Data Lakes and Data Warehouses, discussing their use cases, challenges, and how to choose the best option for your business. Whether you’re looking to enhance analytics, manage diverse data types, or streamline costs, this guide will help you resolve the Data Lake vs. Data Warehouse debate.
Understanding Data Management in Modern Businesses
Data is growing exponentially, and managing it efficiently is essential. Companies rely on data not only for day-to-day operations but also for long-term strategic decision-making. Thus, organizations need effective solutions to store, process, and analyze both structured and unstructured data. This is where Data Lakes and Data Warehouses come into play.
What is a Data Lake?
A Data Lake is a large storage repository that holds vast amounts of raw, unprocessed data in its native format. This data can be structured, semi-structured, or unstructured, which provides flexibility for organizations.
Key Features:
- Flexibility: Data Lakes can store data in any format, from structured tables to unstructured media files.
- Scalability: They are highly scalable, allowing businesses to store massive amounts of data.
- Schema-on-read: Data is structured when accessed or queried, making it highly versatile.
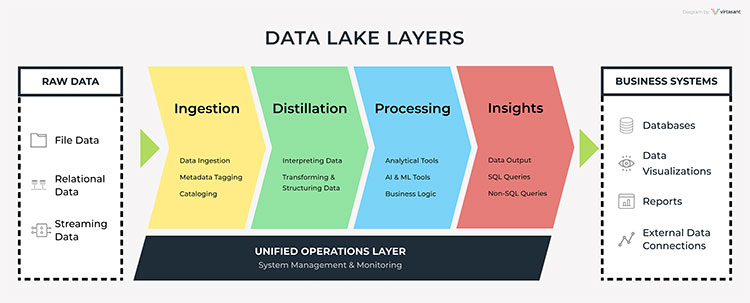
What is a Data Warehouse?
A Data Warehouse is a system that aggregates structured data from various sources, organizing it for analysis and reporting. The data is transformed and structured before storing, making it efficient for business intelligence tools and dashboards.
Key Features:
- Structured Data: Data Warehouses store structured, organized data.
- Schema-on-write: Data is processed and transformed before being stored, optimizing it for fast querying.
- Optimized for BI: Data Warehouses are specifically designed for business analytics and reporting.
Data Lake vs. Data Warehouse at a Glance
Aspect | Data Lake | Data Warehouse |
---|---|---|
Data Structure | Unstructured and semi-structured | Structured |
Schema | Schema-on-read | Schema-on-write |
Storage Cost | Lower costs, stores raw data | Higher cost due to data processing |
Use Case | Machine learning, AI, IoT | Business intelligence, reporting |
User Access | Data scientists, engineers | Business analysts, executives |
Performance | Slower querying, exploratory | Faster querying, optimized for BI |
When to Choose a Data Lake
Data Lakes excel in scenarios where flexibility and scalability are needed for unstructured or semi-structured data. Common use cases include:
- Machine Learning & AI: Data Lakes can store vast amounts of training data for machine learning models.
- IoT Data: Ideal for processing real-time data from IoT devices.
- Exploratory Analytics: Data Lakes allow for flexible exploration of data without the need for strict schemas.
When to Choose a Data Warehouse
Data Warehouses are optimal for businesses that need structured data for regular reporting and analytics. Some common use cases include:
- Business Intelligence: Dashboards, financial reports, and operational metrics can be easily derived from structured data.
- Historical Data Analysis: Organizations looking for trends and performance metrics over time benefit from a Data Warehouse.
- Retail & eCommerce: Retailers tracking inventory, sales, and customer behavior use Data Warehouses for daily reports.
Hybrid Approach: Leveraging Both Data Lake and Data Warehouse
Businesses can also opt for a hybrid solution, utilizing both a Data Lake and a Data Warehouse to optimize their data strategy.
- Raw Data in Data Lake: All incoming raw data (unstructured and structured) is stored in the Data Lake.
- Processed Data in Data Warehouse: Structured data that needs to be queried frequently or used for reporting is moved to the Data Warehouse.
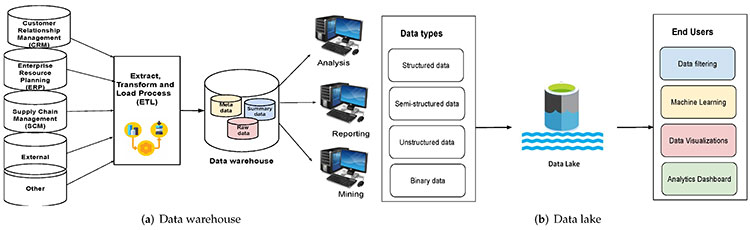
Factors to Consider When Choosing Between Data Lake and Data Warehouse
-
Data Types
If your business handles primarily structured data (e.g., customer orders, financial transactions), a Data Warehouse may be the better choice. Conversely, if you deal with unstructured data like videos, audio, or IoT sensor data, a Data Lake will be more appropriate.
-
Performance and Speed
Data Warehouses offer faster querying and are optimized for Business Intelligence, while Data Lakes may offer slower performance due to the need for data processing during querying.
-
Costs
Data Lakes are generally more cost-effective for raw, unprocessed data, while Data Warehouses come with higher costs due to processing and storage overhead.
-
Data Governance and Security
While both systems require stringent governance and security practices, Data Warehouses tend to offer more built-in security features, whereas Data Lakes need more advanced governance controls.
Key Characteristic: Data Lake and Data Warehouse
Characteristic | Data Lake | Data Warehouse |
---|---|---|
Storage Capacity | Nearly unlimited | Limited by structured design |
Data Type | Unstructured, semi-structured | Structured |
Processing Method | Schema-on-read | Schema-on-write |
Cost | Lower, more scalable | Higher, more processing needed |
Governance | Advanced governance needed | Well-established security |
Key Challenges of Data Storage
Data storage is becoming increasingly complex as organizations grow and collect data from multiple sources. Whether you’re using a Data Lake, Data Warehouse, or a hybrid system, there are several key challenges that you may encounter.
-
Data Volume
The sheer amount of data businesses collect can pose significant challenges. Data is generated from multiple sources such as customer interactions, IoT devices, or digital platforms, and it requires substantial storage space. Cloud-based solutions like Amazon S3 or Azure Data Lake Storage are often necessary to scale up quickly, but costs can add up over time.
-
Data Quality
In a Data Lake, data is stored in its raw form, which can often be incomplete, inconsistent, or even inaccurate. Without proper management, poor data quality can lead to unreliable insights. Data Warehouses require data cleansing during the ETL process, but even this can be labor-intensive if the source data is messy.
-
Data Governance and Security
With data governance regulations like GDPR and CCPA becoming increasingly strict, businesses need to manage who has access to what data. Data Lakes pose additional governance challenges due to their lack of inherent structure, making it harder to apply consistent rules across large, unstructured data sets. Data Warehouses offer better security but at a higher cost due to their strict organization.
-
Data Silos and Integration
In businesses using both Data Lakes and Data Warehouses, data can become siloed, with different departments or systems accessing isolated portions of the overall data pool. This hinders a unified view, impacting decision-making. Integration of different data storage systems requires careful planning and robust architecture.
-
Costs and Optimization
As data grows, so do storage costs. Data Lakes are typically more affordable since they store raw data, but Data Warehouses are expensive due to the need for structured storage and processing. To optimize costs, businesses need to implement strategies for archiving, deduplication, and choosing the right mix of storage solutions.
Conclusion: Finding the Right Data Solution for Your Business
When choosing between a Data Lake and a Data Warehouse, the decision should align with your business goals, data types, and analytics needs. A hybrid approach often works best, combining the strengths of both systems to create a flexible, cost-effective, and high-performance data architecture.
FAQs
Data Lake stores raw, unstructured data, while a Data Warehouse store structured, processed data optimized for querying.
Data Lake is more cost-effective for storing large volumes of raw data, while a Data Warehouse incurs higher costs due to data processing.
Yes, businesses often use a hybrid approach where raw data is stored in the Data Lake and processed data is moved to a Data Warehouse for analytics.